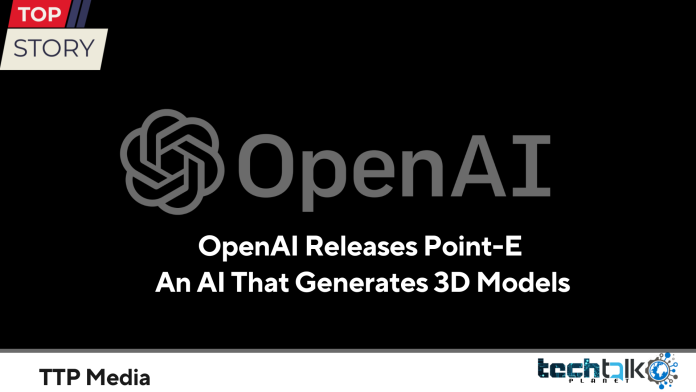
3D model generators may be the next innovation to rock the field of AI. Point-E, a machine learning system that generates a 3D object from a text prompt, was made available to the public this week by OpenAI. A article that was published along with the code base claims that Point-E can create 3D models on a single Nvidia V100 GPU in one to two minutes.
In the conventional sense, Point-E does not produce 3D objects. Instead, it creates point clouds, which are discrete collections of data points in space that reflect 3D shapes; hence, the playful abbreviation. (The “E” in Point-E stands for “efficiency,” as it purports to be quicker than earlier 3D object production techniques.) From a computational perspective, point clouds are simpler to create, but they are currently a major drawback of Point-E because they cannot capture an object’s fine-grained shape or texture.
The Point-E team trained an additional AI system to transform Point-point E’s clouds to meshes in order to get around this restriction. In 3D modelling and design, meshes—collections of vertices, edges, and faces—are frequently used to define objects. However, they make a point in the report that the model occasionally misses specific item details, resulting in blocky or deformed shapes.
Point-E is made up of two models: a text-to-image model and an image-to-3D model, in addition to the mesh-generating model, which is a standalone model. The text-to-image model was trained on tagged images to comprehend the relationships between words and visual concepts, much like generative art systems like OpenAI’s own DALL-E 2 and Stable Diffusion. The image-to-3D model, on the other hand, was taught to effectively translate between the two by being fed a set of photographs coupled with 3D objects.
Point-text-to-image E’s model creates a synthetic rendered item from a text prompt, such as “a 3D printed gear, a single gear, 3 inches in diameter and half inch thick,” and feeds it to the image-to-3D model, which creates a point cloud.
Point-E could generate coloured point clouds that commonly matched word prompts after training the models on a dataset of “several million” 3D objects and related metadata, according to the OpenAI researchers. It’s not flawless; occasionally Point-image-to-3D E’s model cannot interpret the image from the text-to-image model, leading to a shape that does not correspond to the text prompt. Even so, the OpenAI team claims that it is orders of magnitude faster than the prior state-of-the-art.