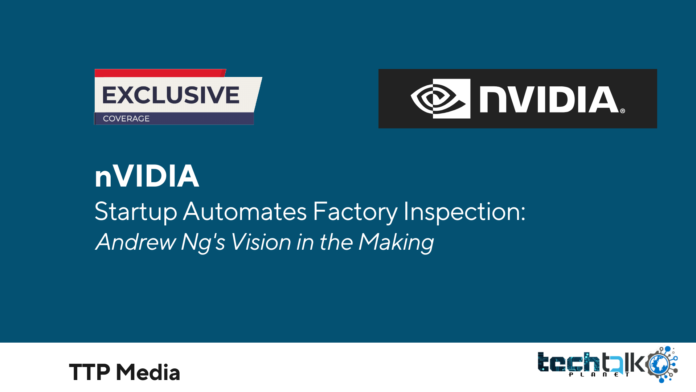
Customers are taking to Ng’s notion of data-centric AI, which is built into Landing AI’s products and accelerated using NVIDIA GPUs.
Landing AI, a computer vision company, has a unique selling point: its co-founder and CEO is a tech rock star.
Andrew Ng made a name for himself at Google Brain by demonstrating how deep learning could spot cats in a sea of photos with astonishing speed and precision. Later, he launched Coursera, which has approximately five million students enrolled in his machine learning courses.
Today, Ng is most recognised for his ideas on data-centric AI, which contends that enhancing AI performance now necessitates a greater focus on datasets rather than neural network model refinement. LandingLens, the flagship product of Landing AI, is built on this principle.
Foxconn, StanleyBlack&Decker, and automotive producer Denso are among the companies that employ Landing AI, which was founded in 2017. Deep learning has been used by them and others to increase efficiency and cut expenses.
A Problem with Classification
One of the first to try Landing Lens was a semiconductor manufacturer with manufacturing sites all around the world. It aimed to enhance the throughput and yield of the wafers that move chips through its fabs by using deep learning.
“They have a lot of visual inspection equipment on the fab floor that scan wafers at various levels — and they do a decent job discovering abnormalities,” said Quinn Killough, Landing’s customer liaison. “But they didn’t do as well categorizing the things they detected into sorts of faults.”
It has also experimented with a variety of classification software packages, as have many other chipmakers. “However, because the solutions had to be fine-tuned for each product,” Killough, who has a background in computer vision and manufacturing, added, “the investment wasn’t worth it.”
Inspection is now automated thanks to artificial intelligence.
The customer then used LandingLens to implement AI. It’s built to handle the whole MLOps process, from data collection through model training and deployment, as well as the continual process of improving the models, particularly the data, to improve outcomes.
Although the implementation is still in its early stages, the product and its data-centric strategy have already helped the chipmaker save money.
“The project’s lead engineer described deep learning as revolutionary, and he wants to spread it out throughout his facility and convince other factories to adopt it,” Killough added.
Landing AI, a computer vision company, has a distinct selling point: its co-founder and CEO is a tech superstar.
Andrew Ng made a name for himself at Google Brain by demonstrating how deep learning could quickly and accurately spot cats in a sea of photos. Later, he launched Coursera, where his machine learning courses have been taken by over five million people.
Ng is most recognised now for his ideas on data-centric AI, which argue that enhancing AI performance now necessitates a greater focus on datasets rather than neural network model refinement. LandingLens, the main product of Landing AI, embodies this principle.
Foxconn, StanleyBlack&Decker, and Denso are among the companies that employ Landing AI, which was founded in 2017. Deep learning has been used by them and others to increase efficiency and lower expenses.
A Problem With Classification
One of the first to try LandingLens was a chipmaker with manufacturing sites all around the world. It wants to apply deep learning to increase wafer throughput and yield in its fabs.
“They have a lot of visual inspection equipment on the fab floor that scan wafers at various levels — and they do a decent job discovering abnormalities,” Quinn Killough, Landing’s customer liaison, said. “But they didn’t do as well categorizing the things they detected into sorts of faults.”
It, like many other semiconductor companies, has tried a variety of software methods for classification. “However, because the solutions had to be fine-tuned for each product,” Killough, who has a background in computer vision and manufacturing, said, “the investment wasn’t worth it.”
Inspection is made easier by artificial intelligence.
Then, using LandingLens, the consumer used AI. It’s built to handle the whole MLOps process, from data collection through model training and deployment, as well as the continual process of improving the models and, in particular, the data, to improve outcomes.
Although the product and its data-centric strategy are still in its early stages of adoption, they have already helped the chipmaker save money.
“The project’s main engineer hailed deep learning as revolutionary,” Killough said, adding that he wants to implement it throughout his facility and encourage other factories to do the same.
Cloud-based auditors
In a cloud-based service that does inference on hundreds of thousands of photos per day, the chipmaker deployed LandingLens on NVIDIA V100 GPUs.
“At first, we weren’t sure about the throughput capabilities, but it’s evident today that it can manage that and a lot more,” Killough explained.
Users may iterate quickly using the same service, which can train a new classification model in about a minute using roughly 50 fault photos.
“On the training side, it’s vital for our product to feel snappy so our clients can solve problems and test out various solutions,” he added.
Taking Artificial Intelligence to the Limits of Its Applicability
With a new solution called LandingEdge, which is now in beta testing with numerous clients, the business is bringing AI to the production floor.
It uses industrial PCs with NVIDIA Jetson AGX Xavier modules to gather pictures from cameras and execute inference on them. The information gained from this effort is fed directly to the controllers that drive robotic arms, conveyor belts, and other industrial equipment.
“We want to increase quality standards, which will create a flywheel effect for quick and iterative AI processes,” said Jason Chan, LandingEdge’s product manager.
Boosting the Growth of a Startup
Landing AI joined the NVIDIA Metropolis initiative, which is aimed for firms who use AI vision to make places and operations safer and more efficient, to gain early access to the newest technology and experience.
It’s still early days for the startup and data-centric AI, which Ng believes will be one of the most significant technological revolutions of the next decade.
Watch a free GTC session (with registration) where Ng discusses the current state and future of the data-centric AI movement to learn more.